University of Virginia scientists combining artificial intelligence and decades of human research are identifying drugs that could help minimize harmful scarring after a heart attack and improve patient outcomes.
“Many common diseases such as heart disease, metabolic disease and cancer are complex and hard to treat,” said researcher Anders R. Nelson, a computational biologist and a former student in the lab of UVA’s Jeffrey J. Saucerman. “Machine learning helps us reduce this complexity, identify the most important factors that contribute to disease and better understand how drugs can modify diseased cells.”
The new machine-learning tool has found a promising candidate to help prevent harmful heart scarring, something previous drugs did not. UVA researchers say the cutting-edge computer model also has the potential to predict and explain the effects of drugs for other diseases.
“Bridging machine learning with human learning helped us not only predict drugs against fibrosis [scarring], but also explain how they work. This knowledge is needed to design clinical trials and identify potential side effects,” said Saucerman, of UVA’s Department of Biomedical Engineering, a joint program of the School of Medicine and School of Engineering.
Combining Human and Machine Learning
Saucerman and his team combined a computer model based on decades of human knowledge with machine learning to better understand how drugs affect cells called fibroblasts. These cells produce collagen to help repair the heart after injury. But they can also cause harmful scarring as part of the repair process. Saucerman and his team wanted to see if a selection of promising drugs would give doctors more ability to prevent scarring and improve patient outcomes.
Previous attempts to identify drugs targeting fibroblasts focused only on selected aspects of fibroblast behavior. How those drugs work often remains unclear. The knowledge gap has been a major challenge in developing targeted treatments for heart fibrosis.
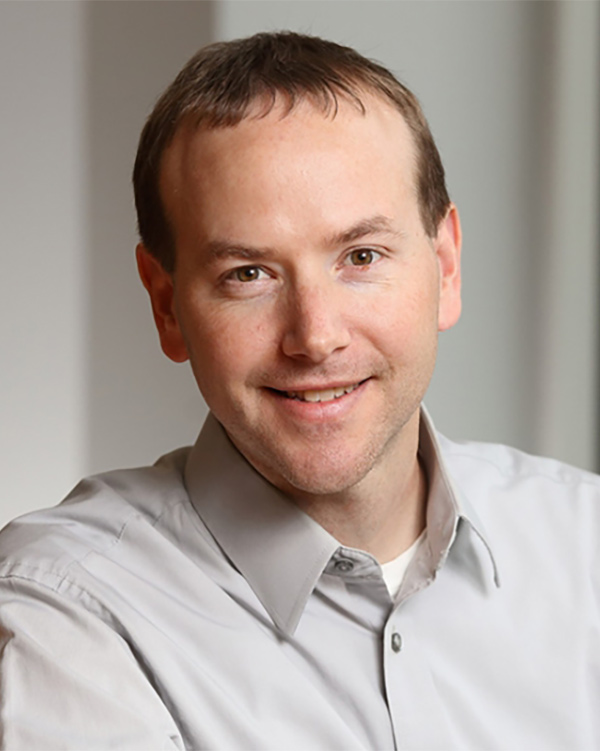
Jeffrey Saucerman is a professor in UVA’s Department of Biomedical Engineering, a joint program of the School of Medicine and School of Engineering. (UVA Health photo)
Saucerman and his colleagues developed a new approach called “logic-based mechanistic machine learning” that not only predicts which drugs may help, but predicts how they affect fibroblast behaviors.
The approach looks at the effect of 13 drugs on human fibroblasts and uses the data to train the machine learning model to predict the drugs’ effects on the cells and cellular behavior.
Additional investigation is needed to verify the drugs work as intended, but UVA researchers say mechanistic machine learning represents a powerful tool for scientists seeking to discover biological cause-and-effect.
“We hope this provides an example of how machine learning and human learning can work together to not only discover, but also understand how new drugs work,” Saucerman said.
Findings Published
The researchers have published their findings in the scientific journal PNAS, the Proceedings of the National Academy of Sciences. The research team consisted of Nelson, Steven L. Christiansen, Kristen M. Naegle and Saucerman. The scientists have no financial interests in the work.
The research was supported by the National Institutes of Health, grants HL137755, HL007284, HL160665, HL162925 and 1S10OD021723-01A1.
To keep up with the latest medical research news from UVA, subscribe to the Making of Medicine blog.
Media Contact
Article Information
May 17, 2025