From music streaming platforms to social media feeds and search engines, algorithms are used behind the scenes to tailor services to the unique preferences of individuals. Though the use of algorithms has been explored in health care since the origins of artificial intelligence, new strides in deep learning methods over the last decade are allowing clinicians to go after mass amounts of data that were previously inaccessible, transforming how doctors and clinical researchers detect, diagnose and treat disease.
In addition to higher data-computing capacities and advanced algorithms, clinicians can now input data through written and spoken words rather than only quantitative lab and imaging results. As they talk with patients about subjective feelings and pain levels, detailed interpretations can be coded to augment “poking and prodding” data collected through sensors, giving machine-learning algorithms a fuller picture. With enough input, algorithms will be able to output a series of patterns which physicians can then use in their clinical practice for better diagnoses and understandings of disease.
“What has happened in the last eight or so years is that new results coming out of these deep learning methods are allowing us to go after hard data problems that weren’t accessible before,” said Don Brown, the University of Virginia’s senior associate dean for research, Quantitative Foundation Distinguished Professor in Data Science and a professor in the Department of Engineering Systems and Environment.
Five years ago, Brown was approached by pediatric gastroenterologist Dr. Sana Syed, who was then working as a gastroenterology fellow in her first year on the UVA faculty.
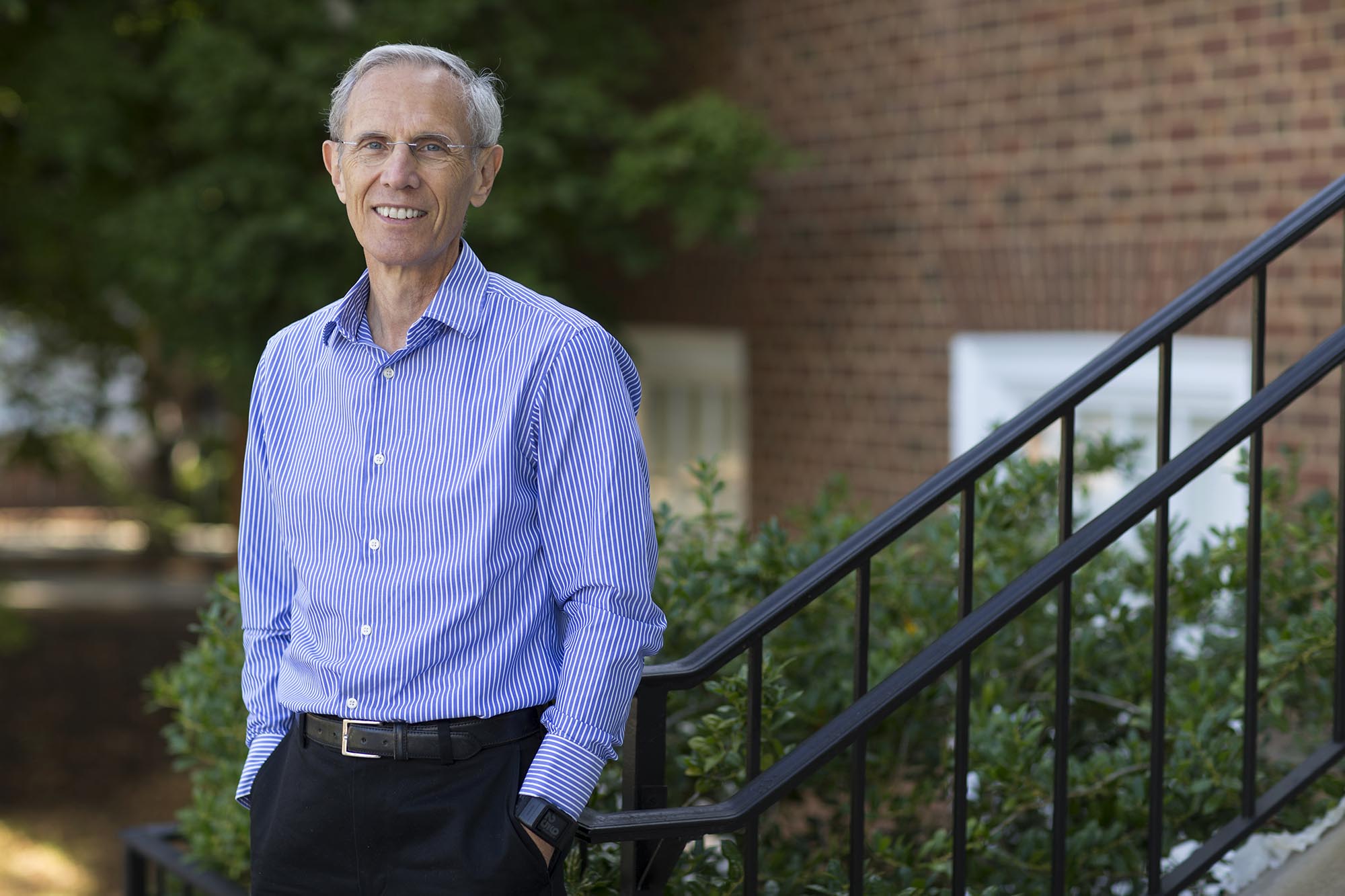
Don Brown is the University of Virginia’s senior associate dean for research, Quantitative Foundation Distinguished Professor in Data Science and the W.S. Calcott Professor in the Department of Engineering Systems and Environment. (Photo by Dan Addison, University Communications)
During her time as a GI fellow, one of the procedures she was trained to do was that of video capsule endoscopy, a procedure that uses a tiny wireless camera to take pictures of your digestive tract. A capsule endoscopy camera sits inside a vitamin-size capsule you swallow. As the capsule travels through your digestive tract, the camera takes thousands of pictures that are transmitted to a recorder you wear on a belt around your waist. Capsule endoscopy helps doctors see inside your small intestine – an area that isn’t easily reached with more-traditional endoscopy procedures. As a fellow, once these videos were downloaded from the recorder, Syed’s role was essentially that of an algorithm. By sifting through thousands of images taken by a video capsule Syed was tasked with tagging any abnormal images, such as polyps, bleeding and ulcers. The tedious task pushed Syed to explore the use of AI as a way of picking up patterns of disease in not just videos from capsule endoscopy but also from other image based diagnostic tools such as in radiology and pathology.
That is when Syed approached Don Brown in a pursuit to intersect big data and medicine. With such an accomplishment, physicians could begin to more quickly and more accurately predict important inflammatory bowel disease outcomes – not only assisting physicians, but also giving patients more peace of mind.
“What is going to happen is that a patient shows up, and their data is plugged into these larger algorithms that are trained to learn off patterns that may be representative of you. Then you will be able to predict a specific patient’s outcome,” Syed said. “The idea is that you will be able to get more tailored data and have the ability to give specific risk percentages.”
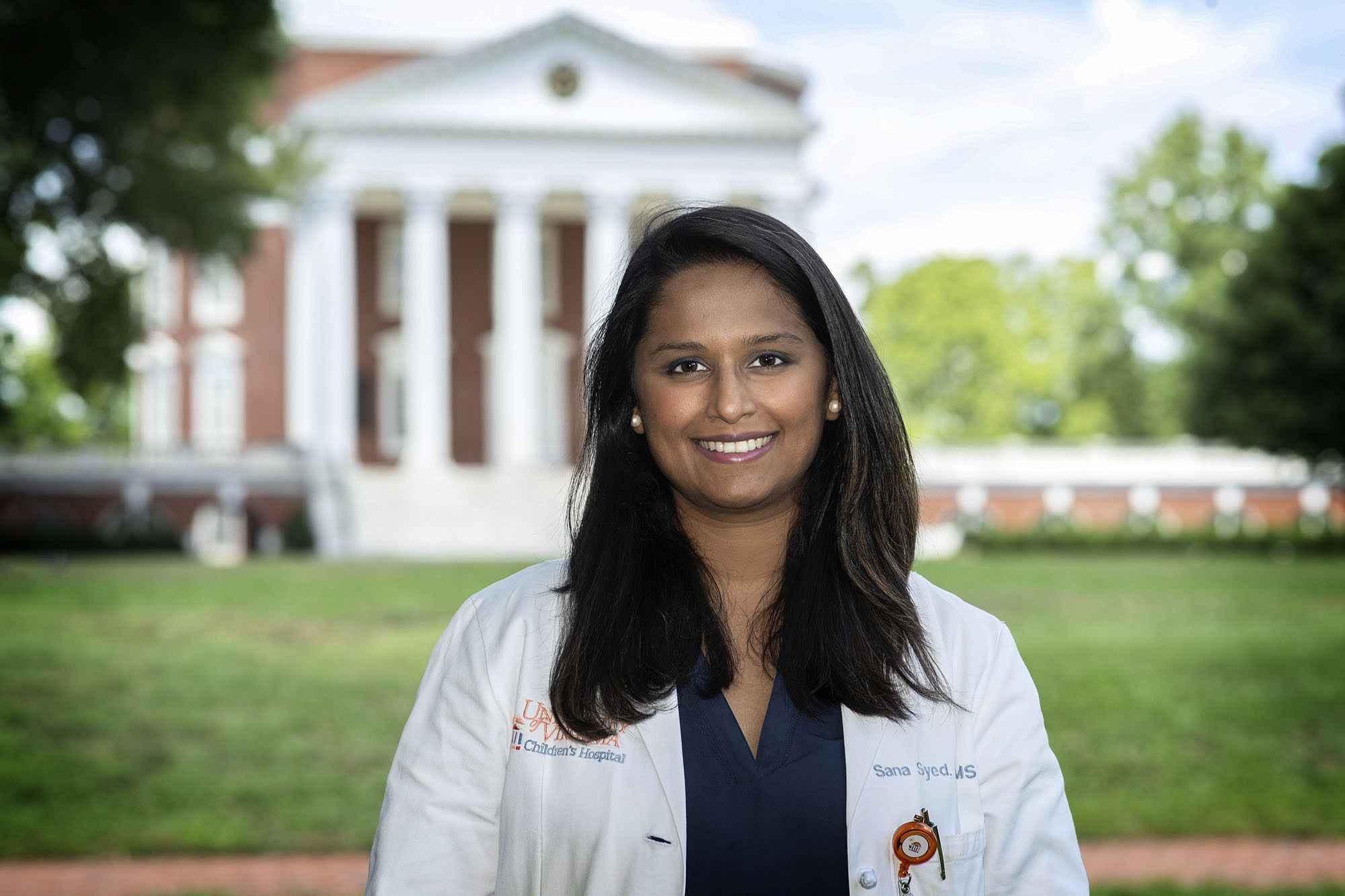
“A lot of this cross-thinking happens when there is open access to data, but that has to be cleaned and sorted and thoughtfully put out there,” said pediatric gastroenterologist Dr. Sana Syed. (Photo by Dan Addison, University Communications)
In addition to identifying patterns in environmental enteropathy, Syed is researching the effectiveness of AI in other inflammatory bowel diseases, such as Crohn’s and celiac disease. According to Syed, algorithms that predict scar tissue development in Crohn’s patients, for example, or individualized risk percentages for thyroid disease and diabetes in celiac patients, would be game-changers in determining more accurate diagnosis and likelihood of disease, allowing clinicians to develop targeted medications and treatments.
“That is the thinking we are moving toward: Let’s not just try to stop the disease; we want to prevent it and cure it. That is the goal.”
To ensure that AI in medicine is effective, Brown said that data scientists and clinical researchers must be aware of inherent biases prevalent in smaller data sets that are many times missed when that data is generalized.
“Algorithms work in a way that gets it the highest reward, and if it can get that reward through a biased set of data, it will use it,” Brown said. “You have to make sure that … you are giving [the algorithm] a fair cross section of data so that it can give you results that are truly answering the question that you are after.”
For Syed, one of the biggest issues lies in the lack of publicly available data sets. When determining patterns in celiac patients, Syed and her team parsed through 2,000 to 3,000 electronic health records to collect data from the desired population. Instead of recreating the wheel by labeling hundreds of thousands of biopsies, Syed hopes to gather medical information through large industry partners such as Takeda, a R&D-driven global biopharmaceutical company.
“A lot of this cross-thinking happens when there is open access to data, but that has to be cleaned and sorted and thoughtfully put out there,” Syed said.
Once data is collected and confirmed to be bias-free, the final step is ensuring interpretability. When many clinicians do not have a background in data science, the only way to cross the disciplinary boundary is to ensure that the data is communicated effectively, which is a responsibility that falls back on the data science community.
“At the heart of this is making it interpretable to the clinicians so that they get what it is that the system is telling them and how it can be used, and what worth it is,” Brown said. “And then, they can look at individual patients and decide what makes sense.”
Machine learning algorithms are being used across medical disciplines throughout the world of health. Within UVA Grounds, algorithms are being used to predict the effects of cardiac disease treatments, understand health conditions through smart watches in UVA’s Link Lab, and analyze real-time diabetes-monitoring data, to name a few. As Syed, Brown and other researchers continue to evolve their AI capabilities, clinicians will begin to transform the ways in which they can accurately predict, determine and treat disease.
“The more I have learned about [big data], the more I have understood its potential impact in medicine across all specialties,” Syed said.
Media Contact
Article Information
February 18, 2022
/content/medicine-meets-big-data-clinicians-look-ai-disease-prediction-and-prevention