Mohamad Alipour envisions a day when an inspector will send a robot to shoot thousands of images of hard-to-reach portions of a bridge and then feed them to a computer, which will review them and flag areas of potential danger.
The engineering graduate student’s work exists at “the intersection of machine learning, visual recognition and civil engineering,” he said.
Alipour, a civil engineering Ph.D. candidate, is part of civil engineering professor Devin Harris’ Mobile Laboratory for Rapid Evaluation of Transportation Infrastructure, also known as “the MOB Lab.”
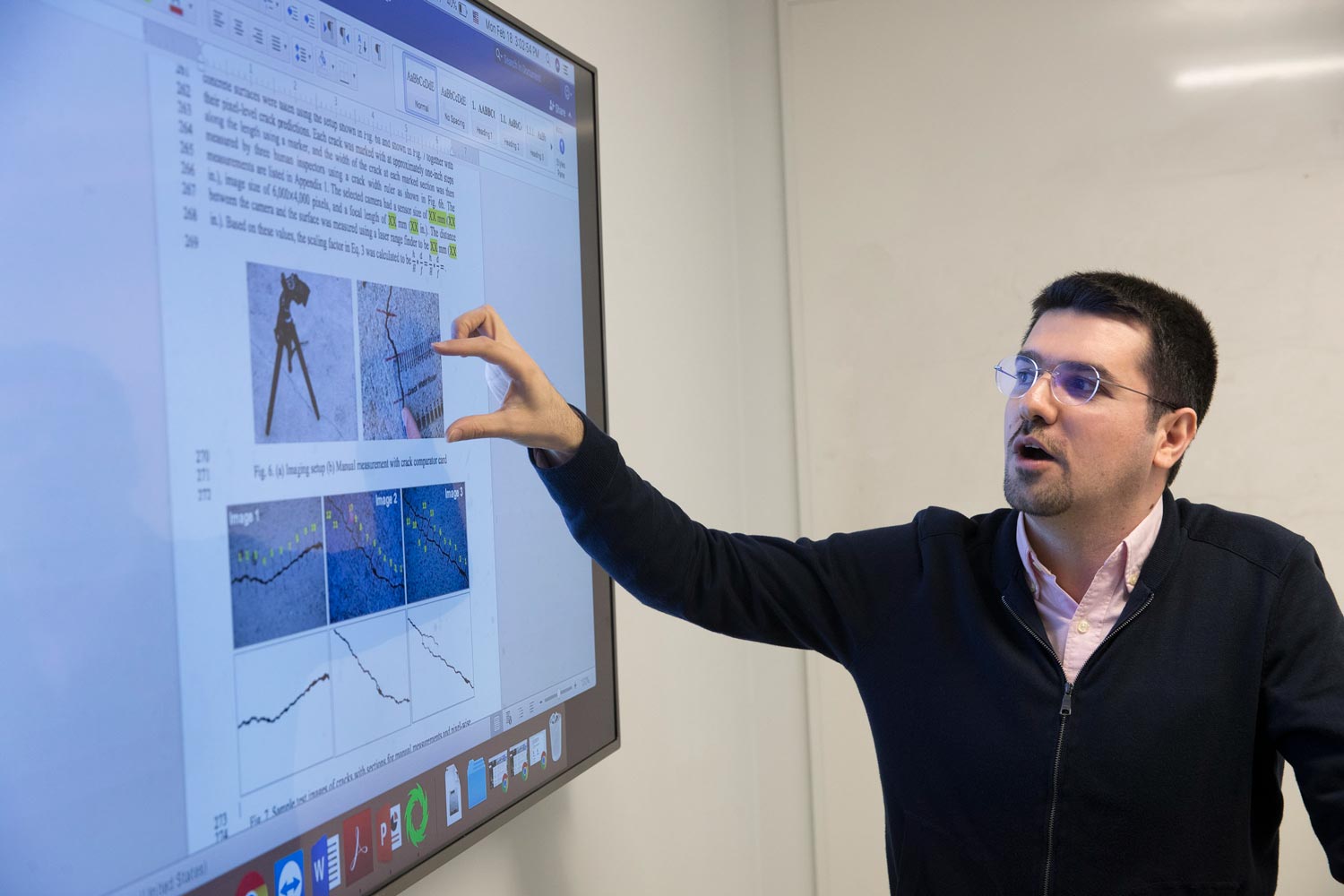
Computers need to be taught what a crack looks like, as Mohamad Alipour demonstrates.
“The group works on a variety of topics related to smart cities and the built environment, with a number of students pursing topics in the data analytics space,” said Harris, an associate professor in the Department of Engineering Systems and Environment and director of the Center for Transportation Studies. “Many of our efforts do center on bridges, but this is mainly due to my own background in bridge evaluation. However, the work is applicable to other infrastructure.”
Alipour, who came to the U.S. from Iran in 2014 to pursue his Ph.D., said robots and computers have the potential to greatly enhance the state of the art in structural inspections.
“The current state of practice in civil engineering is based on routine inspections that are mandated by federal and state transportation agencies that are manual and labor-intensive,” he said. “Professional inspectors are sent to the bridges on a biennial basis to look at everything, manually and in person. This is time-consuming and can be dangerous because people sometimes have to traverse or climb the bridge to be able to get close to everything. You have to get arms-length close to the structure to do a good job of inspecting.”
Autonomous robots can scan bridges and take thousands of photos and videos searching for cracks and flaws. The question then becomes how to use the huge amounts of collected data.
“You cannot have someone sit down and look at all of this imagery to find cracks; that is going to be very time-consuming and resource-intensive,” Alipour said. “So there need to be computer models that process the video and detect features of interest. That is where deep learning and machine learning come in.”
“Machine learning” refers to a series of methods and algorithms used to teach a computer to do things that humans normally do, and “deep learning” is a subset under the machine learning umbrella. Many of these approaches leverage artificial neural networks, which create a set of models based on structures inspired by the human brain and the corresponding biological neural network. This approach creates the foundation for basic functions, such as image recognition using a computer.
“We are training models to be able to detect features of interest in imagery coming from a variety of sources, including drones,” Alipour said. “For example, cracks are of interest to us, and so are corrosion, section loss, and many other modes of damage and failure in transportation infrastructure.
“At the same time, we also want the computer to determine the context of damage by identifying the structural components. Are we looking at a pier in a bridge, a beam, or a foundation? Another characteristic that is important is the size of the crack. Because the wider a crack is, the more important it can be.”
To create their visual recognition models, Alipour and the other researchers feed the computer thousands of images of structures such as bridges with the cracks clearly marked, so the machine can determine or “learn” what cracks on a surface look like.
The researchers take high-resolution images, zoom in on the image to the pixel and then have experts manually find and delineate the crack on a touchscreen. This serves as the training data for the deep learning models.
“As more and more images are added to the training regime, the model gets better and better,” Alipour said. “We perform expert annotations at the pixel level which enables us to quantify the defects and measure their intensity.”
To distinguish, Alipour said they also add intentionally distracting images such as expansion joints in concrete sidewalks, marked “not cracks,” so the algorithms can distinguish between a crack in a road surface and crack-like features, such as a joint between two paving panels.
“You cannot do this with only a few images because the computer is not going to find the pattern,” Alipour said. “That is how deep learning works.”
“Mohamad was certainly the catalyst for much of the work we are doing now within the group, and we have some staggered overlap within the group, so I anticipate that this work will continue after he graduates,” Harris said.
Machine learning also would allow what Harris and Alipour refer to as “citizen engineers,” where people could take pictures with their mobile devices of condition features in urban infrastructure such as potholes, or what they perceive is a structural problem, and send them to a computer that would analyze them using the trained visual recognition models.
“Imagine in the future, we would have a map of Charlottesville with red zones where there are a whole lot of reports from citizens in the form of pictures that show potholes in a part of the road,” Alipour said. “Multiple people have taken those pictures and they can be used by the city or the authorities to pinpoint where they need to work. This is a different type of application from the drone.”
While Alipour is working on visual recognition models, robotic application is also a future component of the project and he is developing collaborations with researchers across the Engineering School, largely through the Link Lab in Olsson Hall.
“The Link Lab is certainly an environment where I interact with students from disciplines such as computer science, electrical engineering, systems engineering and mechanical engineering, and we get into exciting conversations with these people,” Alipour said. “These discussions have certainly helped a lot and saved a lot of time. It gives you ideas and then you can run with those ideas.”
Harris said he has had a positive experience since joining the Link Lab a year ago.
“I think that the benefits realized from being part of the Link Lab have included a heavier focus on advancing technology in my core domain space and the opportunity to engage with researchers from other domains with more ease,” Harris said. “I think that the experience for the students in my group has been the same, as we are all surrounded by researchers doing a variety of things.”
Alipour’s work has been recognized beyond his immediate colleagues. He has received an $8,000 O.H. Ammann Fellowship from the Structural Engineering Institute of the American Society of Civil Engineers for his research and to recognize what he can contribute to structural engineering.
“I feel that part of the reason they gave this award to me is that I am very passionate about these ideas,” Alipour said. “I might have done a good job of reflecting that passion in my statement.”
Alipour likes it when people appreciate his ideas and his work, noting that two years ago he was awarded first place for presenting his ideas in UVA’s fifth annual “three-minute thesis” competition for graduate students.
“It felt as if people really like these ideas,” he said. “The feedback has been positive from both the civil engineering community and the general public. Civil engineers appreciate the idea, but it was particularly encouraging to see the interest from a general audience from different fields.”
Media Contact
Article Information
March 12, 2019
/content/grad-student-trains-robots-computers-find-flaws-bridges-and-buildings