Since its founding three years ago, one of the top goals of the University of Virginia’s Data Science Institute has been to help create opportunities for cross-Grounds collaboration in “big data” research.
Through a grant from the Jefferson Trust, the institute, in collaboration with the Office of the Vice President for Research, has inspired graduate students in diverse disciplines to work together on big ideas involving real-world problems, and to attack those problems with data-driven solutions.
Several projects have come to fruition through a competitive process that advances some of the most innovative ideas. Their leaders are named Presidential Fellows in Data Science, in recognition of the support by the UVA President’s Office.

“This fellowship provides a truly rare and valuable opportunity,” said Don Brown, director of the Data Science Institute. “At very few universities would you find graduate students in systems engineering and psychology working on improved understanding of suicide risk, or graduate students in English and psychology exploring the language of climate change.”
This kind of “cross-cutting, impactful work” is typically available only to those obtaining post-Ph.D. appointments in research centers and institutes that are focused on particular problems, he said, adding that alumni of the one-year fellows program have produced research papers and participated in the generation of new proposals that sprung out of their fellowship activities.
“We are very proud of these students, their faculty advisers and their achievements,” Brown said.
Below are descriptions of some of the most recent projects, begun in 2015. To learn more about these and other projects, and the graduate students who lead them, click here.
Data-Driven Design of Movement and Sound
Led by Lin Bai, a Ph.D. candidate in electrical and computer engineering, and Jon Bellona, a Ph.D. candidate in music
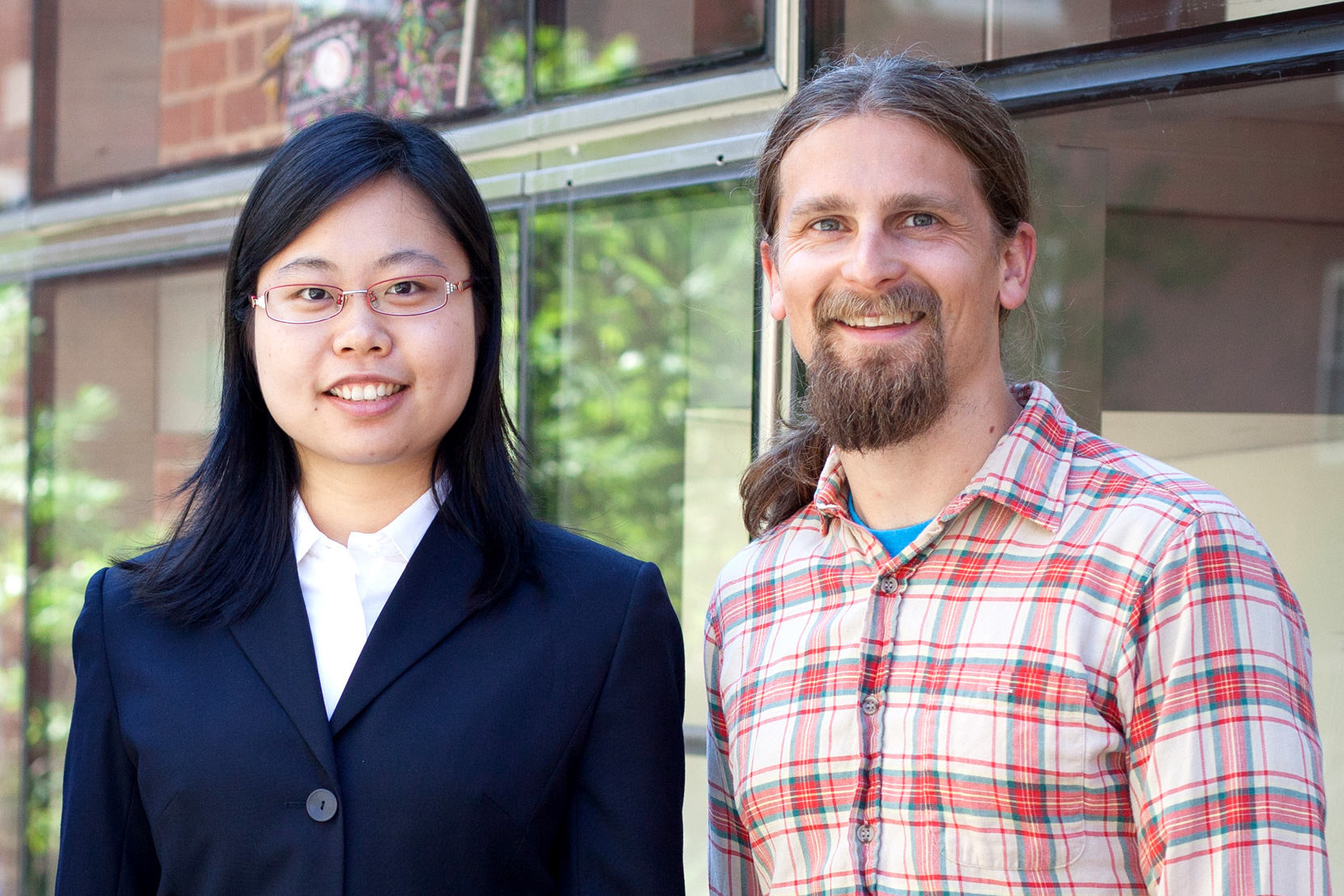
Lin Bai, Ph.D. candidate in electrical and computer engineering, and Jon Bellona, Ph.D. candidate in music. (Photos courtesy of the Data Science Institute)
Formal project description: Our team is working to improve perceived variation in robotic movements. By capturing and analyzing human vocalizations created in response to simulated motions, the project will develop robotic movements that are synchronized with perceptually designed sonifications as a way to make robotic movements appear more expressive, increasing the level of human perception of quality in robotic movement.
What’s really going on: How do you tell if someone is calm, excited, or sad? Humans communicate nonverbally through the expressive qualities of their movements. We also communicate through non-verbal aspects of our voice. The pitch, loudness and speed of our voices can tell listeners about our emotional state. What if robots could also move expressively? Some members of our team and others are working on this problem; however, there are practical limitations. Our work, a collaboration between roboticists and musicians, aims to give robots an expressive “voice” so that robots will be able to better interact with and work alongside humans in various settings such as manufacturing, health care or the home.
Big data’s role: We recorded musicians making expressive sounds to match the qualities of expressive movements. Using signal processing and statistical tools, we are analyzing various qualities in these sounds in order to understand how sonic features map to features of movement. We will validate our findings through a large study to test whether these mappings lead to more accurate perception of expressive qualities in robotic movement.
Applying Machine Learning to Text Communications to Model Suicide Risk in Real Time
Led by Jeffrey Glenn, a Ph.D. candidate in psychology, and Alicia Nobles, a Ph.D. candidate in systems and information engineering
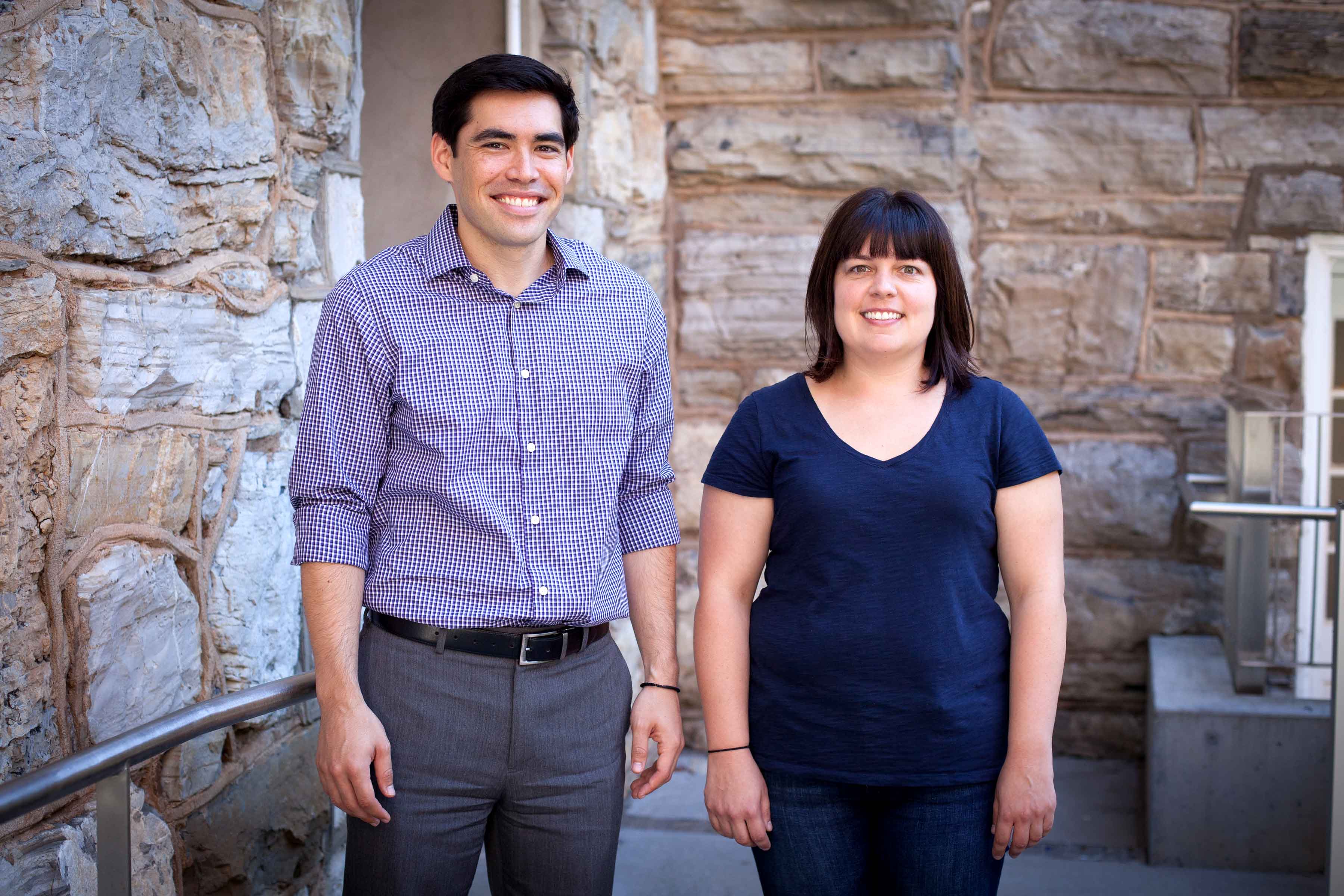
Jeffrey Glenn, Ph.D. candidate in psychology, and Alicia Nobles, Ph.D. candidate in systems and information engineering.
Formal project description: Our team is working to improve objective assessments of suicide risk by examining electronic communications of people with a history of suicidal thoughts and behaviors to identify communication patterns indicative of heightened suicide risk.
What’s really going on: Suicide is the second-leading cause of death among young adults, but the challenges of preventing suicide are significant because the signs are often invisible. Research has shown that clinicians are not able to reliably predict when someone is at greatest risk. Our project asks, “Can big data techniques help us see what we as humans cannot? And specifically, can personal electronic communication, such as phone text messages, inform us about one’s suicide risk?” This project is a direct response to the urgent need for novel, data-driven tools to objectively assess acute suicide risk.
Big data’s role: Our study focuses on building a multimodal dataset, including a clinical interview of mental health history, text messages, call history, emails, social media data and web browsing activity from individuals with a history of suicidal thoughts and behaviors. Big data techniques, such as natural language processing, machine learning and data visualization, will be applied to identify unique patterns of communication that occur in advance of a suicide attempt. These techniques can increase visibility of subtle clues in communication, indicating when someone is in a suicidal state and allowing clinicians to more objectively evaluate when patients are especially vulnerable to hurting themselves.
Partisan Speech and Climate Change: a Toolkit for Detecting Deliberate Diction
Led by James Ascher, a Ph.D. candidate in English, and Bommae Kim, a Ph.D. candidate in psychology
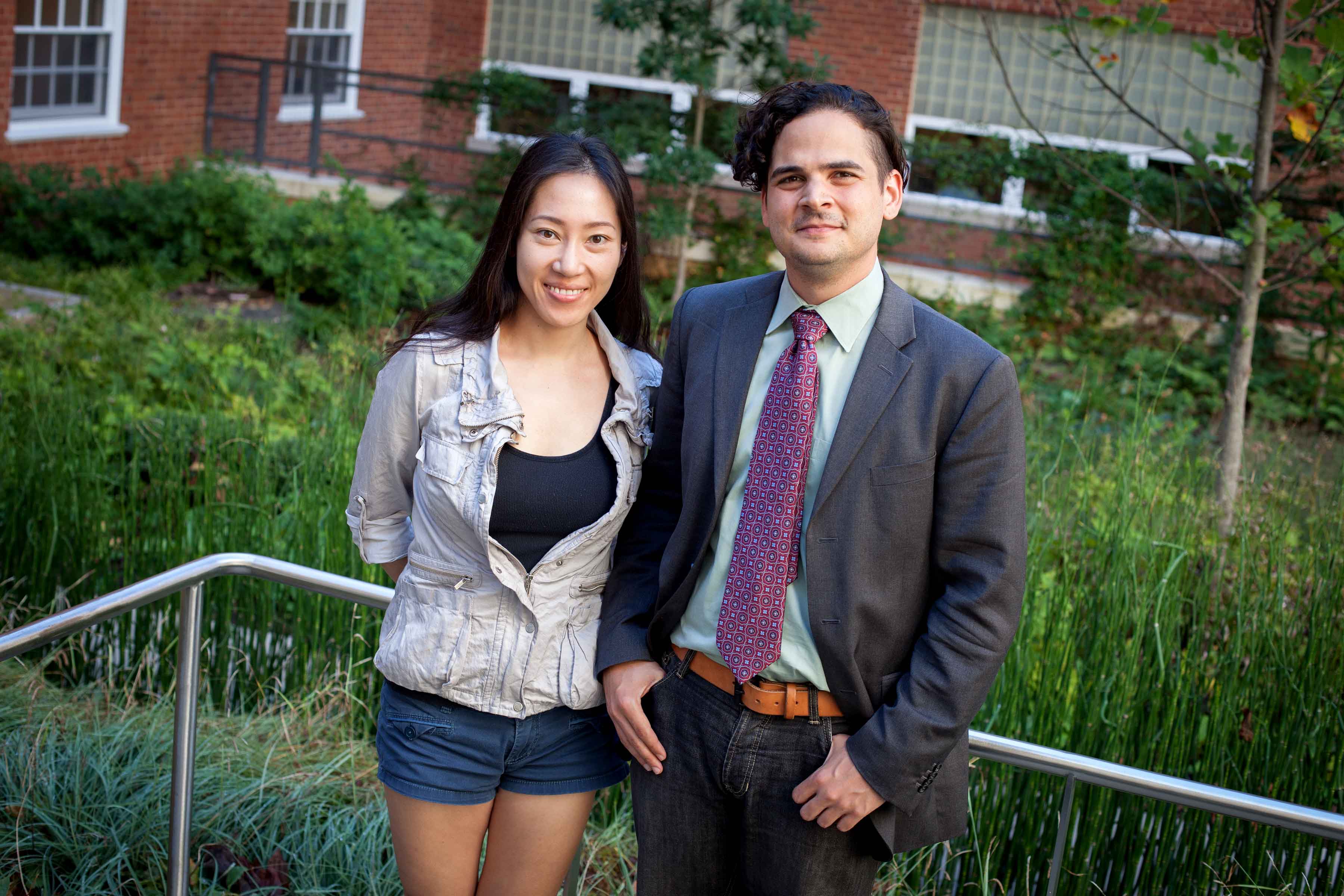
Bommae Kim, Ph.D. candidate in psychology, and James Ascher, Ph.D. candidate in English.
Formal project description: Our project is aimed to understand political speech regarding climate change using natural language processing and machine learning of a corpus of edited texts. However, in the process we uncovered larger problems regarding partisan speech, repeatability and credibility of knowledge. In understanding the language used in Congress that argued against the growing scientific consensus regarding climate change, we begin to understand something much bigger – we traced the diction and rhetorical patterns used by representatives, experts and senators to deny climate change, but these patterns turned out to be much older.
What’s really going on: By the end of our project, we had developed a toolkit and tested a series of exercises with a first-year writing class that not only demonstrated the particular partisan speech for climate change, but also recreated the process that developed that partisan language. Among other things, we developed a classroom technique of “paper dialing,” which used a classroom of thinkers in a way that parallels formalized and computerized textual analysis. As we developed our techniques, we realized that the problem was one of access to knowledge, and began carefully documenting the tools to make them available to a smart college student without supervision.
Big data’s role: Our collaboration continues to develop this toolkit and generalize it. We can teach computers and freshmen how to detect the diction and language of climate change and explain how that language came to be the way it is through focus groups and dialing sessions. But we’re working on documenting and packaging our tools so that the same techniques could be applied to any political controversies in ethical and responsible ways. Our original work is becoming an example and a case study for a larger method for analyzing partisan speech that we plan to make available to any citizen-scientist who wants to study how things are being discussed.
Multi-Agent Modeling and Analysis of Large-Scale Brain Networks with a Big fMRI Data Set
Led by Marlen Gonzalez, a Ph.D. candidate in psychology, Shize Su, a Ph.D. candidate in electrical and computer engineering, and Qiannan Yin, a Ph.D. candidate in statistics
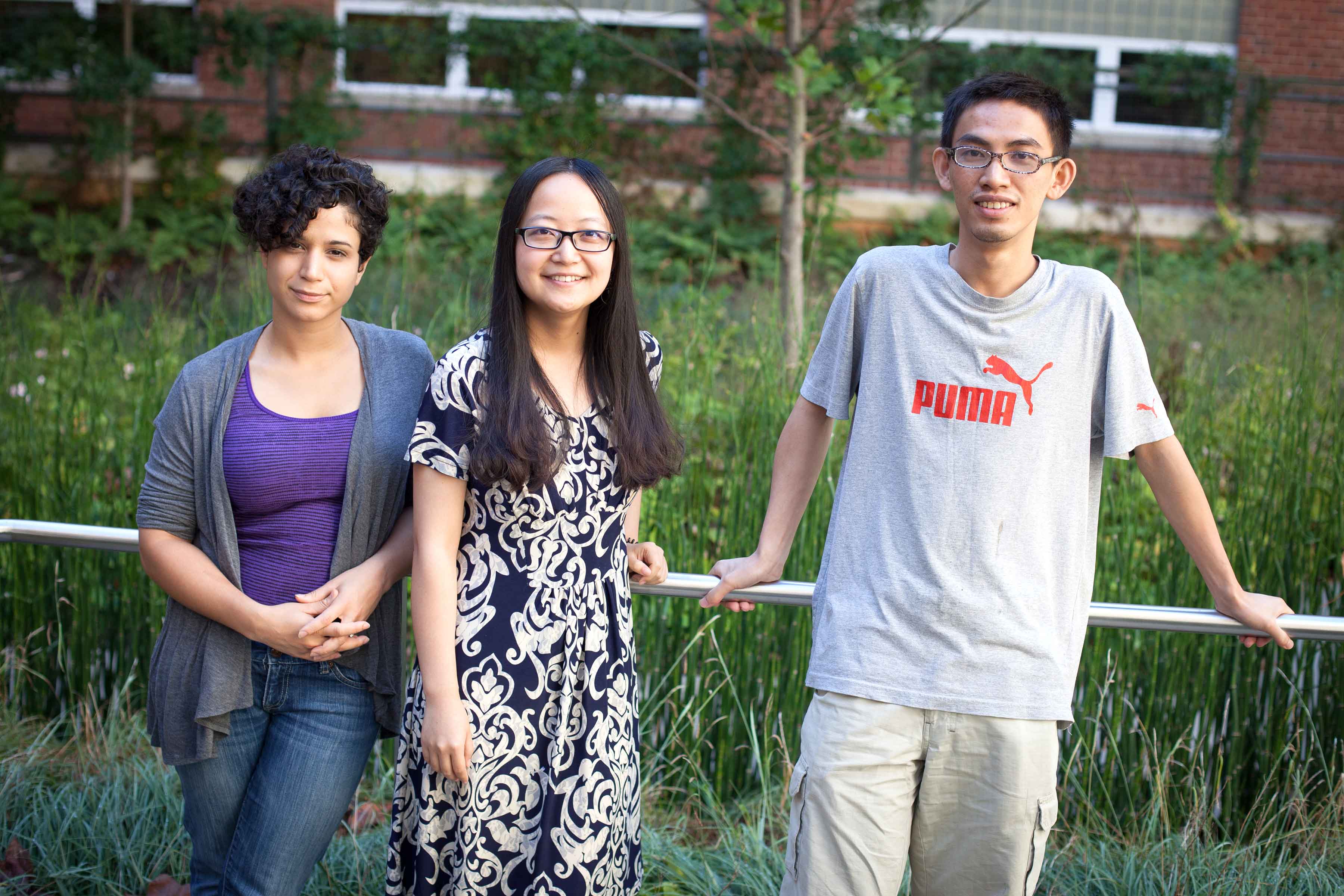
Marlen Gonzalez, left, Ph.D. candidate in psychology, Qiannan Yin, center, Ph.D. candidate in statistics, and Shize Su, Ph.D. candidate in electrical and computer engineering.
Formal project description: This project will analyze large-scale brain functional networks involved in the social regulation of emotion using both statistical methods and engineering tools. Using data from a social support functional neuroimaging study, we will model the brain as a dynamic network with nodes referring to different brain regions and lines representing the interactions between each pair of brain areas.
What’s really going on: The main objective is to identify and discover some important patterns of the complex brain functional network that are valid for psychological interpretation, via sophisticated analysis of the large fMRI (functional magnetic resonance imaging) data collected from psychological experiments. This will deepen understanding of the complexity of brain networks to improve human brain health, add to the literature at large on the physiological effects of social support and derive new methods to learn more from pre-existing fMRI large datasets.
Big data’s role: A large fMRI data set of more than 100 participants was collected and the data re-analyzed, involving about 40 billion brain interactions – which is massive. This would then be multiplied by three experimental conditions and then by 100 subjects. Therefore, the amount of data is beyond “big data.” We are developing various novel, efficient techniques to significantly reduce the computational burden at the price of only some minor information loss. Without such techniques, the computation for pattern extraction from such a large brain network would take many years even on a powerful computer cluster, or otherwise would be infeasible.
Media Contact
Article Information
November 30, 2016
/content/data-science-presidential-fellows-bring-life-data-research-and-vice-versa