How do job-seekers determine the right career fit? How can they project employment decisions into the future?
These are questions Denis Nekipelov wants to answer. An associate professor of economics at the University of Virginia, he is working with a researcher at talent recruitment agency Riviera Partners on a National Science Foundation-backed research program called a “convergence accelerator.” The NSF-funded program requires academic and private sector partners to collaborate on a practical project.
“One of the goals of this program is to make useful things faster,” Nekipelov said. “It doesn’t apply to everything, but I think some technologies can be fast-forwarded to produce something that is consumer-ready.”
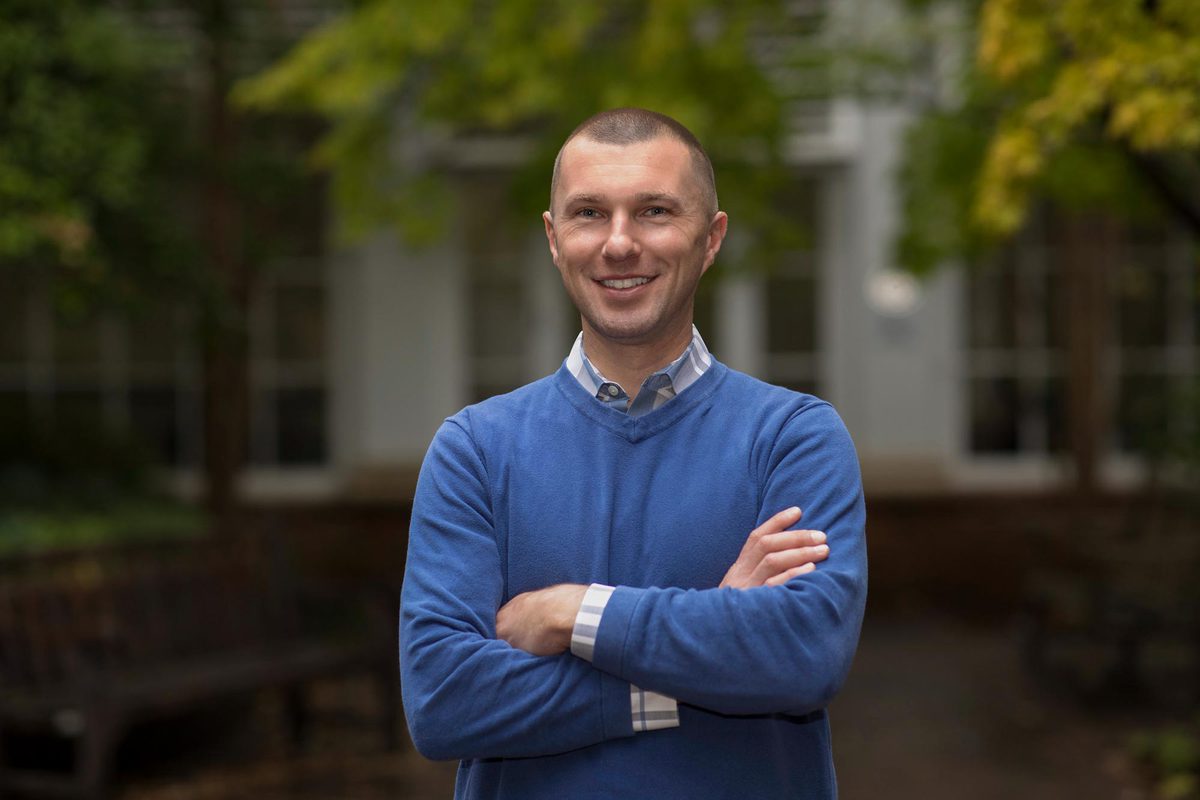
Denis Nekipelov started in mathematics and physics, and then shifted a short distance to economics. (Photo by Dan Addison, University Communications)
Nekipelov is concentrating on how to fill jobs more accurately and faster, particularly in the high-technology industry, which is flush with cash and expanding rapidly. In that sector, companies are chasing the same pool of potential employees, engineers and programmers.
“As a result, anyone who is remotely qualified would observe a bunch of offers coming their way every time,” Nekipelov said. “And one of the biggest problems in this circumstance is people not knowing how their choices today may result in their choosing something based on very superficial qualities.”
Nekipelov thinks that engineers could take a job with a large company such as Google or Microsoft and find themselves working a succession of similar projects that will not help them advance their careers – in essence, not getting 10 years’ experience, but one year’s experience 10 times.
“Within these big companies, it is very difficult to move up the ladder because there are 10,000 people like you who are competing for the same position that is on the level above,” Nekipelov said. “In many cases, instead of going for the brand name and the free food, it is better to take something in a smaller company where you are going to be growing, and the scope of the projects will fast-track this individual to some really big projects by the end of his or her career.”
Nekipelov and his research partner, Yiqing Wang of Riviera, are developing an algorithm to determine how a candidate’s career path will look in five years and 10 years, based on the choices he or she makes today.
“I think just visualizing what a career path would look like is potentially going to be really groundbreaking,” Nekipelov said. “And the nature of the Silicon Valley market is that the people you see in the press and of whom you know the stories are essentially the superstars. But we only have one Mark Zuckerberg; we don’t have 100 of him, so looking at the superstars, everyone expects to become CEO in three years. That forms unrealistic expectations – and frustration as well.”
Nekipelov said the end result of his research may tamp down expectations and give potential employees a more realistic view of what they need to do and how to prepare for their career.
“You should not be expecting to be running the company next year,” Nekipelov said. “And to actually run the company, you have to go through this set of steps. You should be growing your team at this pace; you should be taking this job over that job. I think it is going to create a lot more sustainable medium for people to progress along their careers.”
Nekipelov also sees this research project as a way to make the economy run more smoothly. reduce disruptions
“From the purely economic perspective, what happens when people make decisions without good information is that the quality of matches between the companies and the workers are just not very good,” Nekipelov said. “And so, if you find yourself in an environment that is not a good match, that is bad for both sides. The company does not get a good worker and the worker is not satisfied and so it is not a good fit.”
Creating an algorithm that predicts a reasonable career arc provides a company and a prospective employee with information Nekipelov is convinced will improve the quality of the match.
“Once there is a good and comprehensive way of communicating information, you can improve the market by redesigning it,” he said. “Maybe the next step is to actually create a matching platform that is going to be market-scale. Before going there, you need to establish communication between both sides. That is where our project is going.”
But how much communication do you need to have a good fit? Nekipelov said his team is working on a model that has about 300 factors on each side, for the employer and the potential employee, but he does not yet know how many boxes have to be checked on each side to have a good match. The desires of the employer and the potential employee must be translated into a common language of mathematics.
“This is something we are currently calibrating,” he said. “That is part of the science part of this project. We have now a pretty good idea of what the feature space looks like. We have the full text of someone’s résumé, and now we need to convert it into something that is mathematically studiable.”
The research project has two phases. The current, nine-month first phase is funded at $730,000. For the second phase, Nekipelov and Wang must pitch the project to a panel of judges from the National Science Foundation. If approved for the next rounds, they will have about 30 months in which to complete the research.
“The NSF is keeping track and making sure we are not going to get stuck at any point,” Nekipelov said. “We have dedicated coaches who are assigned to all the teams and have the experience of commercializing academic technologies, essentially making things that work from academic research.”
The research, based on an idea Nekipelov and Wang devised while they were working together, is partly personal for Nekipelov, who said he is frustrated by his friends’ uninformed decision-making.
“We were discussing something along the lines of this technology, because it comes from our mutual frustration at seeing how people get stuck,” Nekipelov said. “It was both our idea. It’s not the most ground-breaking idea.”
Nekipelov thinks communication is the key to interactions.
“I have worked with a few companies in the past, and one of the biggest things I have learned from market design is that you can create a beautiful mechanism, something theoretically sound, in which the engineering works beautifully. But if the users do not understand it – if you do not communicate very well with the users, if you don’t explain to them how to form expectations – then everything is going to crash and burn,” he said.
Nekipelov is Russian, born as the son of two engineers at a submarine base in far northern Russia. The base is now closed and his parents live in Moscow. He received bachelor’s and master’s degrees in applied physics and mathematics from the Moscow Institute of Physics and Technology, and then a master’s degree in economics from the New Economic School in Moscow.
He shifted from math and physics to economics after performing a mathematical analysis of the Russian tax system in the 1990s, trying to find the sweet spot in a progressive tax system that ranged from 10% to 38%.
“My role was as the Excel spreadsheet person,” he said. “I was an undergraduate student at the time and I was trying to figure out what is going to be the rate where, if nothing changes and we are going to replace that highly progressive system with a flat tax, what is going to be the rate of the flat tax where the revenues are not going to be impacted?”
He pegged it at 13% and he found a new career.
He continued his education, earning a Ph.D. in economics from Duke University, and then followed his adviser to Stanford University. He worked for Microsoft for several years; taught at the University of California, Berkeley; then returned to Microsoft – where he met Wang, who was “director of monetization” for the technology conglomerate. Nekipelov came to UVA in 2014, but stayed in touch with Wang.
To Nekipelov, economics shares a lot with physics.
“The distance between them is not that great, which actually surprised me,” he said. “In some sense, physics is something that tries to model the world, in physics we’re just trying to model the physical world. So there is a set of empirical observations that we are going to turn into laws or some kind of characterization that are going to be mathematical and theoretical; economics works in a similar way.
“And while it is much more difficult to predict humans than to predict atoms or molecules, there are some general guiding principles. People tend to have preferences, and they typically don’t change very quickly, so within the periods of stability, take the past choices and turn them into predictions about future choices in the short run, and if preferences change, we can have models for exactly how they change again based on empirical observations.”
Media Contact
Article Information
December 3, 2019
/content/economist-pursues-tool-help-job-seekers-find-right-fit-faster