Two students with similar credentials emerge from a high-stakes job interview with a top management consulting firm. Both feel they established strong rapport, answered all behavioral interview questions thoroughly and aced the case interview.
One applicant gets the job. The other does not.
Why?
Did the company make the right choice?
Those complicated, sprawling questions are at the heart of new research by a trio of University of Virginia Ph.D. students – two from UVA’s Darden School of Business and one from the School of Engineering and Applied Science – who are using new data science tools to answer questions related to what makes a job interview successful for both the candidate and the recruiter.
The research project was recently awarded a Presidential Fellowship in data science from the UVA Data Science Institute, one of six projects chosen from across the University. Each pairs data science experts with experts in other fields to develop novel tools and techniques to address significant issues and solve problems.
The project – Improving Recruiting & Mitigating Bias: Using People Analytics to Improve the Way Organizations Identify, Attract, Develop, and Retain Talent – brings together Brendan Boler, a Darden Ph.D. candidate in the leadership and organizational behavior area; Travis Elliott, a Ph.D. candidate in the strategy, ethics & entrepreneurship area at Darden; and Tianlu Wang, a Ph.D. candidate in the School of Engineering and Applied Science’s Department of Computer Science. Faculty advisers on the project include Darden professors Bobby Parmar and Jared Harris and School of Engineering professor Vicente Ordonez-Roman.
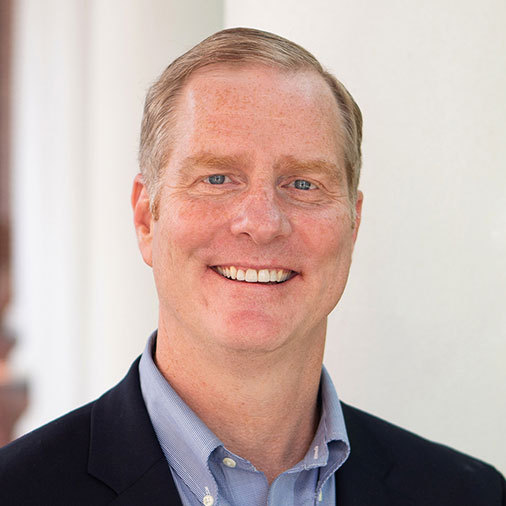
Brendan Boler, a Darden Ph.D. candidate in the leadership and organizational behavior area. (Contributed photo)
Boler, who has taught courses related to management consulting and career strategy at the McIntire School of Commerce, previously worked at the Darden Career Development Center, where he developed an up-close perspective on professional interviews from both the recruiter and applicant points of view.
“I became fascinated with the interview/evaluation process for hiring,” said Boler. “It’s almost like dating. You have a limited amount of time, and you’re being evaluated on multiple fronts. Both your analytical skills and your interpersonal skills are being assessed. And like dating, the evaluators are often overconfident in their abilities to pick the right people.”
Boler also draws an analogy to the sports world, noting the legions of NFL talent evaluators who passed on New England Patriots quarterback Tom Brady, who eventually fell to the 199th pick in the draft. Were the scouts evaluating the right variables?
Are recruiters?
While UVA’s Data Science Institute has become the most recent source of funding for the project, initial funding came when Boler, working with his dissertation committee, was able to win a $60,000 “Three Cavaliers” grant through the UVA Office of the Vice President for Research. The grants were created to encourage collaboration across UVA’s academic disciplines and schools. Boler’s committee consists of three professors from three UVA schools: Parmar from the Darden School, Eileen Chou from the Frank Batten School of Leadership and Public Policy and Gary Ballinger from the McIntire School of Commerce.
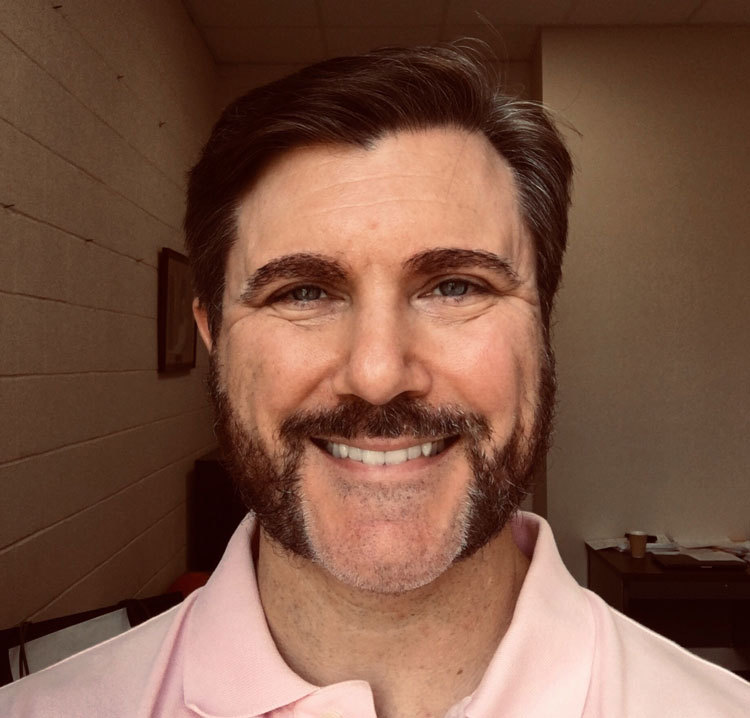
Travis Elliott, a Ph.D. candidate in the strategy, ethics & entrepreneurship area at Darden. (Contributed photo)
“Without their support and encouragement, this ambitious project would have not gotten off the ground,” Boler said.
In total, the two programs will provide nearly $200,000 in funding for the project, Elliott said. The team is nearing completion of the data collection phase and soon will begin the coding and analytics stage.
The project is expected to produce valuable insights for applicants hoping to land their dream jobs and recruiters aiming to find the right candidates.
Mining Data from 200 Interviews to Answer One Burning Question: What Separates Candidates?
Interviews with consulting firms represent a particularly rich vein for new data-gathering, due to their complexity. These interviews typically including an introductory rapport-building stage, a behavioral interview and a case interview involving a complex problem to be analyzed.
There is surprisingly little peer-reviewed research that incorporates an evaluation of the case interview component, Boler said. Working with Elliott and Wang, the trio devised a project that involves collecting data from more than 200 students from six top business schools who were invited to interview at major consulting firms.
In addition to familiar variables like demographics and academic credentials, the researchers are collecting data from a psychological survey and recording interviews, then using cutting-edge text and video analysis tools to build novel data sets and consider the material in new ways. The researchers also track real-world recruiting outcomes of the interviewees, homing in on what matters for a successful recruiting effort.
“It’s taken a lot of work, but we’ve built a rich data set from a concentrated yet diverse group of top-tier candidates from elite schools,” said Elliott. “The logistical planning behind getting access to these candidates and visiting schools, reserving rooms, compensating participants, collecting data on paper and online, completing surveys, and recording hour-long interviews in high definition with over 200 people has been immense but also extremely rewarding.”
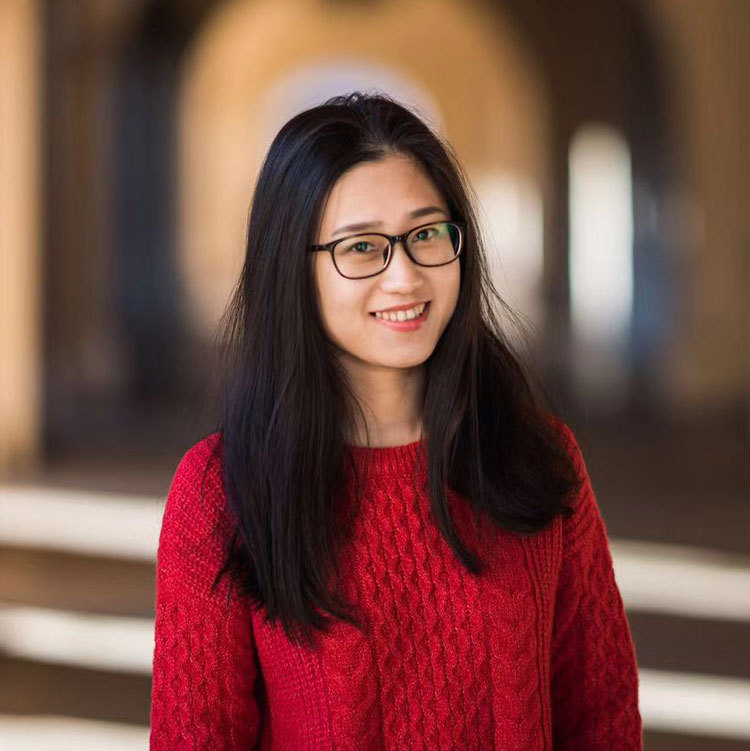
Tianlu Wang, a Ph.D. candidate in the School of Engineering and Applied Science’s Department of Computer Science. (Contributed photo)
When completed and fully analyzed, Elliott said the team hopes to have a clearer understanding of what separates high-quality candidates from one another, as well as how researchers and recruiters can identify unconscious or implicit bias in the recruiting process and learn how to mitigate it.
“Data science opens up opportunities to create variables that haven’t existed before,” said Elliott. “We can look at factors and combinations of factors we haven’t been able to analyze before and find relationships that we haven’t been able to see before in our research.”
For Wang, the project has been an opportunity to take her computing- and machine-learning prowess into new and unexplored territory.
“My research has focused on leveraging computing power to analyze large data sets of text and images at the intersection of computer vision and natural language processing,” said Wang. “I also am interested in exploring topics concerning fairness and accountability like reducing bias in machine learning applications.”
Elliott said the Presidential Fellowship in Data Science committee took note of just how ambitious the project was, and how determined the team of Ph.D. candidates is to see it through.
“We want recruiting organizations, candidates and schools to be more successful in their recruiting outcomes,” Elliott said. “Despite the competitive nature of recruiting and the advanced science involved in the project, we have to remember that this work ultimately is about people. We want to make a positive impact on individual lives and on organizations.”
Media Contact
Article Information
May 31, 2019
/content/using-data-science-determine-why-one-job-candidate-beats-out-another