Star Wars fans rejoiced in 2019 when the creators of “Star Wars: The Rise of Skywalker” made true-to-life scenes of the late actress Carrie Fisher by taking their cue from Derpfakes, one of the first YouTubers to use deep machine learning to produce phony but realistic-looking videos and images.
Other “deepfake” videos of celebrities and politicians have caused amusement and raised alarm in equal measure. Recent examples include the entertainment news site Collider’s deepfake of a celebrity roundtable that seemed to include director George Lucas and actor Ewan McGregor; a Tom Cruise impersonator’s series of video shorts that garnered millions of views on TikTok, Twitter and other social networks; and comedian Jordan Peele’s lip-synched video of former President Barack Obama – itself a warning about the technology’s misuse.
Though occasionally amusing, the fake videos are certainly misleading and can even be dangerous. A team of University of Virginia School of Engineering undergraduate students has responded by creating an innovative way to prevent and uncover deepfake images, videos and other manipulations online.
This spring, the team earned the top prize in the iDISPLA University Adversarial Artificial Intelligence / Machine Learning Challenge organized by the Greer Institute for Leadership and Innovation on behalf of Nibir Dhar, chief scientist for the U.S. Army Night Vision and Electronic Sensor Directorate.
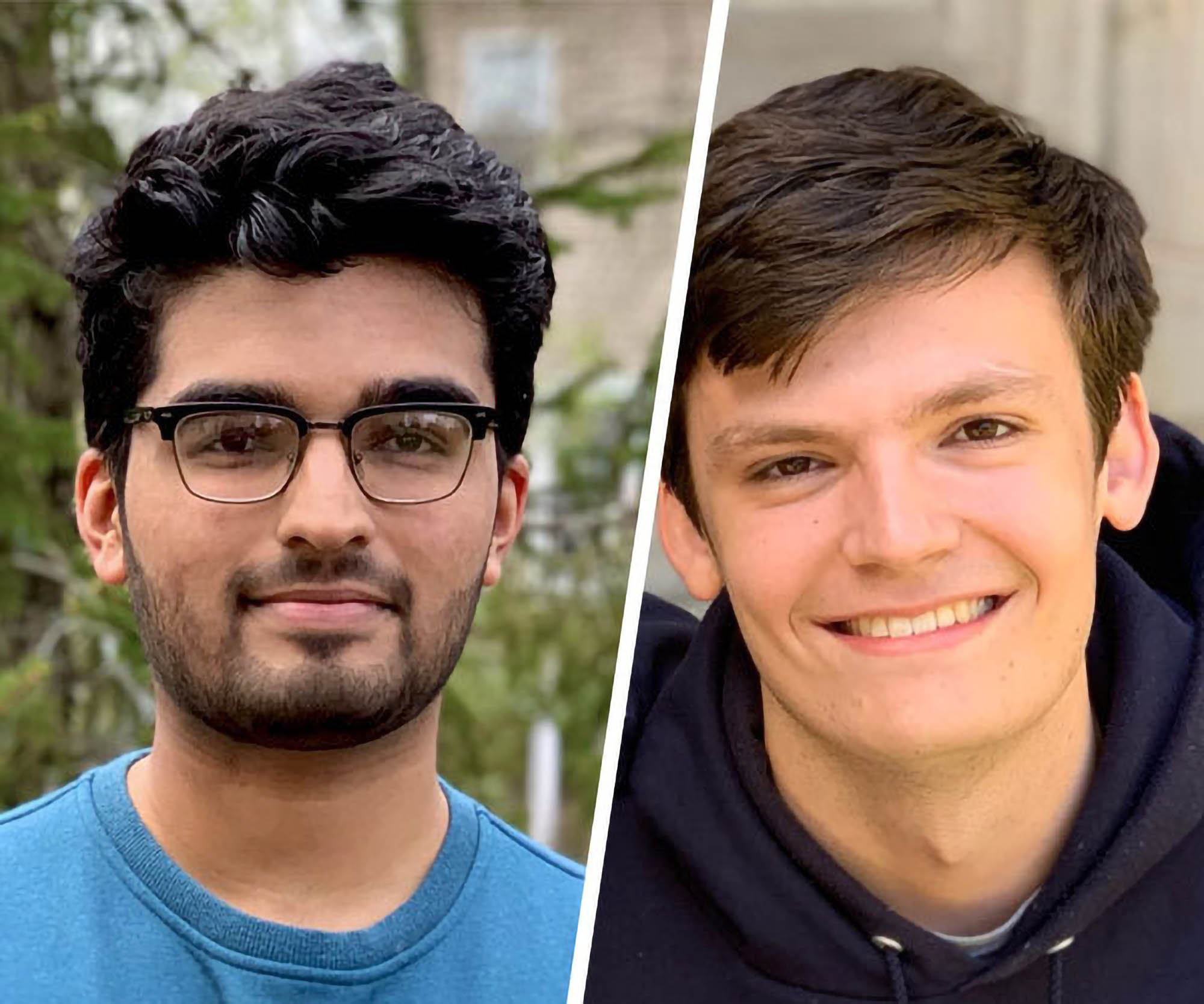
Ahmed Hafeez Hussain, left, and Zachary Yahn have created an innovative way to prevent and uncover deepfake images, videos and other manipulations online. (Contributed photos)
To respond to the challenge, Ahmed Hafeez Hussain, a rising third-year computer engineering and physics major, and Zachary Yahn, a rising third-year computer engineering and computer science major, developed their method to automatically detect and assess the integrity of digital media. Hussain and Yahn received advice and guidance from Mircea Stan, Virginia Microelectronics Consortium professor of electrical and computer engineering, and Samiran Ganguly, a postdoctoral research fellow in UVA’s Virginia Nano-Computing Research Group led by Avik Ghosh, professor of electrical and computer engineering and physics.
“Anyone with an internet connection and knowledge of deep learning can produce a semi-convincing deepfake of politicians and public figures, with the potential to sow mass confusion,” Hussain said. Deep learning is a subset of machine learning based on the human brain.
Social media companies are facing increasing pressure to flag and remove disinformation, including deepfakes. The envisioned service would enable media outlets and platforms to instantly verify whether a reported video is real. Hussain and Yahn presented a novel, end-to-end solution that relies on commonly used blockchain technology (which makes it difficult to hack the system) and cryptographic hashes to rapidly verify potentially deepfaked media on the internet.
How Deepfakes Work
Deepfakes are synthetic media created through the interplay of two deep learning models specifically designed to compete against each other. A generator model is trained to produce replicas while the discriminator model attempts to classify the replicas as either real or fake; they compete until the generator produces plausible replicas, fooling the discriminator at least 50% of the time. This mode of media production is called a generative adversarial network, or GAN. GANs can create artificial images, video and audio that are almost impossible for humans to differentiate from real media.
Generative adversarial networks have beneficial uses; for example, GANs create artificial medical images to train medical students and professionals, generate photo-realistic images from text descriptions, and aid technical design such as in drug development. But they can also be exploited by those with malicious intent: GANs have enabled controversial deepfakes that have found their way to news headlines and social media posts.
The Students’ Solution
The most common approach to combating deepfakes relies on training a “good” machine learning model to identify or disrupt the manipulation. This solution is time- and resource-intensive and unique to an organization’s network, so a systematic and reliable method of identification is a hot research topic.
Some researchers have developed methods to produce images and videos that cannot be easily modified, essentially hardening the target against tampering. This method is promising for newly produced media but does not protect the vast amounts of unfiltered media already published on the internet.
Hussain and Yahn’s approach overcomes these limitations, verifying videos and images on the internet as quickly and accurately as possible through collaboration, only relying on machine-learning algorithms when absolutely necessary.
“All participants benefit from our detection framework, especially media consumers,” Hussain said. “Rather than confining a detecting machine-learning model to a single platform, our framework is implemented onto the web.”
“Whenever a publisher uploads a video to a platform’s website or channel, a video hashing algorithm produces a unique signature for the video; the signature can later be used to determine whether two videos are the same,” Yahn said.
When the image is released, its hash is saved in the publisher’s blockchain – a kind of continuously growing virtual ledger – along with all previous video hashes. “Once something is added to the ledger, it is immutable and publicly available,” Yahn said.
Trust in contributors to the blockchain is an important caveat; the team’s solution presumes that a bonafide news producer manages the blockchain, and only a few verified news sources can contribute to it. Additionally, news consumers have the ability to report a suspected deepfake, prompting the blockchain manager to remove that image from future verifications.
“Ahmed and Zach have proposed a novel and efficient way to connect already effective tools and foster cooperation between media publishing organizations, bringing us one step closer to restoring the veracity of digital media,” Stan said.
As the first-place finisher in the iDISPLA challenge, Hussain’s and Yahn’s team earned a $6,000 award. They will also have the opportunity to hold a virtual lunch-and-learn briefing to Dhar, Melvin Greer, founder of the Greer Institute and head of AI for Intel Corporation, and other members of the AI challenge judges panel.
Hussain and Yahn intend to refine their solution through additional research. Their objectives are to minimize computing resources; further reduce reliance on machine-learning models and apply their solution to “shallowfakes,” a method of manipulating media content using simple video editing software without the use of machine-learning technology and algorithmic systems; and to investigate other data storage functions, for example to reduce the amount of time it takes to process data inputs.
Media Contact
Article Information
June 2, 2021
/content/uva-undergrads-offer-new-approach-detecting-deepfake-videos